Workspan Daily provides news and insights on total rewards topics. Get Workspan Weekly — our email newsletter — and our newest articles will be delivered directly to you.
Workspan Magazine supplies in-depth analysis on pressing issues. Now all digital and delivered with greater frequency — 6x per year!
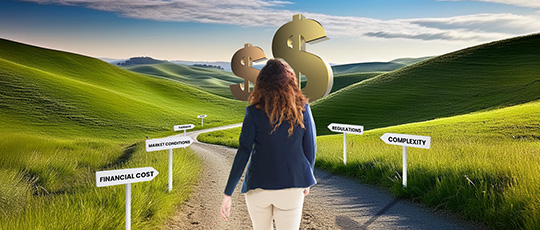
Please try a different combination of filters or categories.
Catch up on past issues of Workspan.
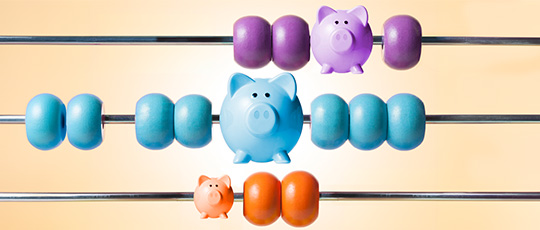
Retirement plan inequity, benefits enrollment and compensation peer groups are among the topics covered in this issue.
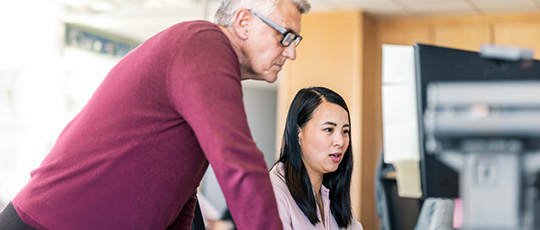
TR pros revamp retention strategies in recognition that baby boomers are a valuable talent pool.
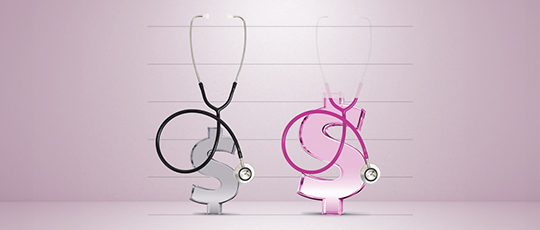
The health care gender gap, paying ERG leaders, instant pay, and more.
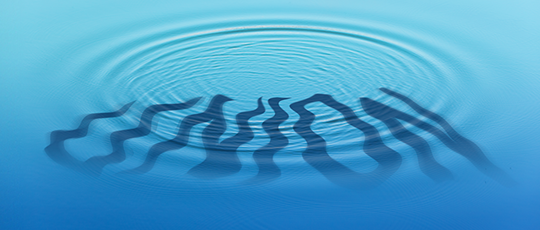
In this issue of Workspan, we examine the union effect on compensation and benefits.